- AlphaSignal
- Posts
- 🥇 This Week's Top 5 AI Papers
🥇 This Week's Top 5 AI Papers
Fresh out the neural network. Our model analyzed and ranked 1000+ papers to provide you with the following summary. Enjoy!
AlphaSignal
Hey ,
Greetings and welcome back to AlphaSignal! Over the past 10 days, a staggering 1682 AI papers have been released. From this wealth of material, we have identified the top 6 papers that stand out from the rest.
This week we are seeing more vision foundation models that follow Meta AI’s SAM from last week. SEEM is already challenging SAM with its flexibility in dealing with the prompts and the capability of class-aware segmentation, not to mention the better naming! Will SAM v2, later on, have even more capabilities than SEEM already has? If yes, what additional capabilities will it include?
NVIDIA’s video LDM is another game changer, as it does not alter any of the parameters from the usual image LDM, making it a perfectly compatible text-to-image model when skipping the newly introduced layers. As in the NLP field, PEFT (parameter efficient fine-tuning) is shining, showing that there is no need to fine-tune all the parameters of the foundation model. As LDMs are widely acknowledged for their efficiency in compute, will the T2V models have a paradigm shift towards latent generative models? Only time will tell.
On the LLM side, methods that do not even require fine-tuning—tool-augmented LLMs—are improving yet again. Chameleon is equipped with even more modules than the previous methods and pushes the performance on general tasks by a large margin. As a fast-emerging research field, how far will these tool-augmented LLMs push the field forward? On the other hand, will these methods alleviate the lack of credibility and verifiability of generative language models? If you have any questions, suggestions, or feedback, please do not hesitate to reply to this email, and we will be prompt in getting back to you.
Let's get into it!
Lior
Here's what’s in today’s summary:
The Wordcloud of 1000+ papers ☁️
Chameleon: Plug-and-Play Compositional Reasoning with Large Language Models
Segment Everything Everywhere All at Once
Align your Latents: High-Resolution Video Synthesis with Latent
Other notable papers
☁️ ABSTRACTS WORLDCLOUD

📄 TOP PUBLICATIONS
Chameleon: Plug-and-Play Compositional Reasoning with Large Language Models
Score: 9.9 • Pan Lu, Baolin Peng, Hao Cheng, Michel Galley, Kai-Wei Chang, Ying Nian Wu

Summary
Chameleon, a remarkable plug-and-play compositional reasoning framework that leverages GPT-4 and seamlessly integrates vision models, web search, and even Python scripts. Built upon the foundation of tool-augmented Language Models (LLMs), Chameleon utilizes natural language planning to retrieve knowledge from the given question, plan for the necessary actions, and generate a coherent answer. While other tool-augmented LLMs exist, Chameleon stands out with its impressive performance, even outperforming state-of-the-art methods like Chain of Thought (CoT) and fine-tuned models such as LLaMa-Adapter in some benchmarks.
At its core, Chameleon comprises a natural language planner (P) and a set of possible usable modules (M). When presented with a query, P selects the appropriate modules from M, invokes them sequentially, and generates the final answer with the final module. Chameleon can be thought of as the generalized version of CoT, with a broader set of viable modules beyond the "Solution Generator" and the "Answer Generator."
In ScienceQA, Chameleon achieves a remarkable 86.54% accuracy, representing a significant boost of more than 11% over the previous few-shot GPT-4 based approach. Similarly, for TabMWP, Chameleon achieves an impressive 98.78% accuracy, a 17.8% improvement over the previous state-of-the-art. With its seamless integration of diverse modules and impressive performance, Chameleon represents a significant advancement in the field of tool-augmented LLMs, holding the potential to unlock new frontiers in natural language processing and reasoning.
Details
Submitted on Apr 20 • Computation and Language • Mathematical Reasoning
Want to promote a product, job, or event to 100,000+ AI researchers and engineers? Reach out here.
Segment Everything Everywhere All at Once
Score: 9.0 • Xueyan Zou, Jianwei Yang, Hao Zhang, Feng Li, Linjie Li, Jianfeng Gao

Summary
A cutting-edge segmentation model, SEEM (Segment Everything Everywhere All at Once), has recently been introduced to the field, boasting even greater capabilities than the recent SAM. While SEEM's evaluation is currently limited to relatively small-scale, well-established datasets like COCO, the model boasts an impressive range of features, including the ability to handle the composition of prompts, memory prompts for enhanced interactive segmentation, and zero-shot class-aware segmentation.
Like SAM, SEEM's architecture incorporates a universal encoder that encodes image-text representations in a shared space, as well as a lightweight decoder that produces masks and class embeddings. This design offers advantages in interactive scenarios, where features can be pre-computed and the decoder can be queried multiple times. Furthermore, visual and text prompts are aligned with mask and class embeddings to improve performance, and at test time, visual/text prompts can be concatenated and fed into the network, even if they were not trained in this way.
Despite its relative novelty, SEEM demonstrates exceptional performance, standing alone as the only model that can effectively handle all types of generic segmentation tasks, including panoptic/instance/semantic, as well as referring segmentation or interactive segmentation. Overall, SEEM represents a major leap forward in the field of segmentation models, with exciting implications for the future of computer vision
Details
Submitted on Apr 14 • Computer Vision and Pattern Recognition • Semantic Segmentation
Align your Latents: High-Resolution Video Synthesis with Latent Diffusion Models
Score: 8.5 • Andreas Blattmann, Robin Rombach, Huan Ling, Tim Dockhorn, Seung Wook Kim, Sanja Fidler, Karsten Kreis
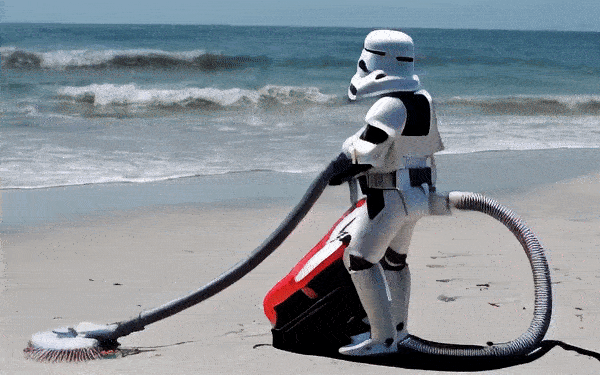
Summary
NVIDIA drops a new text-to-video diffusion model that is based on a pre-trained latent diffusion model (LDM; i.e. stable diffusion). The video diffusion model is constructed by interleaving additional trainable temporal consistency layers as conv3D blocks and temporal attention blocks, while leaving all the original image LDM parameters fixed. The model is capable of 512x1024 resolution driving video simulation, and text-to-video generation of 1280x2048 resolution that spans more than a few seconds with state-of-the-art quality.
On top of the interleaving strategy, the authors additionally fine-tune the decoder that maps the latents back into the sequence of images by leveraging a patch-wise video discriminator. Furthermore, using the same interleaving strategy, the authors also train the temporal upsampling LDM that operates directly on the latents. After the sequence of images are decoded, another spatial upsampling LDM is used to bring the video to the megapixel regime.
The model is versatile in that it directly leverages the off-the-shelf image LDM and does not alter any of the weights, and only requires training of the additionally introduced layers. Moreover, it inherits several advantages of LDMs: video personalization using DreemBooth, and easier conditioning on visual prompts such as bounding boxes.
Details
Submitted on Apr 06 • Computer Vision and Pattern Recognition • Image Segmentation
🏅 NOTABLE PAPERS
Zip-NeRF: Anti-Aliased Grid-Based Neural Radiance Fields
Score: 9.0 • Jonathan T. Barron, Ben Mildenhall, Dor Verbin, Pratul P. Srinivasan, Peter Hedman
AutoTaskFormer: Searching Vision Transformers for Multi-task Learning
Score: 6.4 • Yang Liu, Shen Yan, Yuge Zhang, Kan Ren, Quanlu Zhang, Zebin Ren, Deng Cai, Mi Zhang
Evaluating Verifiability in Generative Search Engines
Score: 6.6 • Nelson F. Liu, Tianyi Zhang, Percy Liang
Thank You